The nature of random errors and sample statistics distribution
Sample statistics distribution >> Random errors >> Measuring social characteristics
Nobody likes to make mistakes, but some mistakes are inevitable!
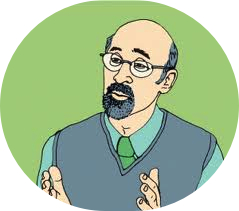
Dembitskyi S. The nature of random errors and sample statistics distribution [Electronic resource]. - Access mode: http://www.soc-research.info/quantitative_eng/3.html
The differences in characteristics of the sample and the population are called representativeness errors. We can distinguish between two types of such errors – systematic and random.
Systematic errors are certain constant biases which are not reduced with the increase in the number of respondents. Random errors are those that change within the laws of probability with the increase in the sample.
Systematic errors can be eliminated by changing the sampling procedure; random errors will always occur, in any sampling survey. Nevertheless, a systematic error is much more dangerous, because: a) it is impossible to estimate; b) it does not decrease with the increase in sample size.
A classical example of the research failure due to the systematic errors is the election poll held by the Literary Digest in 1936. According to its results, Alfred Landon would have won the presidential election in the U.S. Significant if the fact that more than 2 million respondents were selected for the research for the Literary Digest. The actual election was won by Theodore Roosevelt, whose victory was predicted by Gallup and Roper based on a poll of only 4,000 people.
The Literary Digest’s error was the fact that the sampling frame (the part of the population from which the respondents were selected) was represented by the phone books. Telephones in 1936 could be afforded mainly by the wealthier U.S. population, the majority of which was going to vote for Alfred Landon. Consequently, the resulting sample did not reflect all of the U.S. voters, but only their specific group. It is also clear that increasing the sample obtained in this way would not help, as new respondents would also be wealthy Americans.
Systematic errors are certain constant biases which are not reduced with the increase in the number of respondents. Random errors are those that change within the laws of probability with the increase in the sample.
Systematic errors can be eliminated by changing the sampling procedure; random errors will always occur, in any sampling survey. Nevertheless, a systematic error is much more dangerous, because: a) it is impossible to estimate; b) it does not decrease with the increase in sample size.
A classical example of the research failure due to the systematic errors is the election poll held by the Literary Digest in 1936. According to its results, Alfred Landon would have won the presidential election in the U.S. Significant if the fact that more than 2 million respondents were selected for the research for the Literary Digest. The actual election was won by Theodore Roosevelt, whose victory was predicted by Gallup and Roper based on a poll of only 4,000 people.
The Literary Digest’s error was the fact that the sampling frame (the part of the population from which the respondents were selected) was represented by the phone books. Telephones in 1936 could be afforded mainly by the wealthier U.S. population, the majority of which was going to vote for Alfred Landon. Consequently, the resulting sample did not reflect all of the U.S. voters, but only their specific group. It is also clear that increasing the sample obtained in this way would not help, as new respondents would also be wealthy Americans.
Gallup and Roper poll was random in character and reflected all of the U.S. population, which allowed them to make a correct prediction.
But if the systematic errors do not decrease with the increasing number of respondents and the way to eliminate them should be sought primarily in the construction of the sample itself, the random errors comply with the laws of probability and are subject to evaluation. One of their main properties is the fact that they decrease with the increase in the sample size. Let us consider the following example (partly fantastic).
Let us imagine a huge lottery machine with 100,000 balls, in which there are 10,000 balls with number 1, 10.000 – with number 2, 10.000 – with number 3, 10.000 – with number 4, 10.000 – with number 5, 10.000 – with number 6, 10,000 – with number 7, 10.000 – with number 8, 10.000 – with number 9, and 10.000 – with number 10. Provided the lottery machine functions properly, each ball has an equal probability of dropping out (at least in the beginning, but after the balls will start dropping, the probabilities would be very close). Consequently, there is a 10% probability of a ball with any number dropping out (№ 1 – 10%, № 2 – 10%, etc.). But for random errors, any sample capable of fully implementing the model of the population would have 10% of the balls with each number. Of course, such a sample in reality is very rare specifically due to random errors which introduce a degree of mismatch with the population and its model – the random sample.
Below are the data obtained by a computer program simulating the lottery machine described above:
But if the systematic errors do not decrease with the increasing number of respondents and the way to eliminate them should be sought primarily in the construction of the sample itself, the random errors comply with the laws of probability and are subject to evaluation. One of their main properties is the fact that they decrease with the increase in the sample size. Let us consider the following example (partly fantastic).
Let us imagine a huge lottery machine with 100,000 balls, in which there are 10,000 balls with number 1, 10.000 – with number 2, 10.000 – with number 3, 10.000 – with number 4, 10.000 – with number 5, 10.000 – with number 6, 10,000 – with number 7, 10.000 – with number 8, 10.000 – with number 9, and 10.000 – with number 10. Provided the lottery machine functions properly, each ball has an equal probability of dropping out (at least in the beginning, but after the balls will start dropping, the probabilities would be very close). Consequently, there is a 10% probability of a ball with any number dropping out (№ 1 – 10%, № 2 – 10%, etc.). But for random errors, any sample capable of fully implementing the model of the population would have 10% of the balls with each number. Of course, such a sample in reality is very rare specifically due to random errors which introduce a degree of mismatch with the population and its model – the random sample.
Below are the data obtained by a computer program simulating the lottery machine described above:
The number of ball |
The number of dropped balls after 25, 50, 75 and 100 lottery machine runs |
|||
25 |
50 |
75 |
100 |
|
№1 |
20% |
18% |
14,6% |
13% |
№2 |
8% |
4% |
8% |
8% |
№3 |
16% |
12% |
12% |
11% |
№4 |
4% |
6% |
6,6% |
7% |
№5 |
8% |
10% |
12% |
10% |
№6 |
4% |
14% |
10,6% |
12% |
№7 |
16% |
14% |
10,6% |
10% |
№8 |
8% |
8% |
8% |
8% |
№9 |
8% |
4% |
4% |
8% |
№10 |
8% |
10% |
13,3% |
13% |
Max deviation |
10% |
8% |
6% |
3% |
Mean |
4,92 |
5,18 |
5,25 |
5,48 |
If there were no random errors, then after the first 25 balls dropped out, the distribution for any ball would be 8% and 12% (and not 10%, because the result of dividing 25 by 10 is not an integer), after 50 balls dropped out it would be 10% for each ball, after 75 balls – 9.3% and 10.7% for any ball, after 100 – again 10%.
But as we can see, random errors occurred at each of the four stages. At the first stage, the most frequently dropped ball is number 1, and the maximum deviation from the true value is 10%. At the second stage, the maximum random error is also observed for the ball with number 1, but becomes a little lower – 8%. At the third stage, the maximum error is observed for the ball with number 9, which dropped out in only 4% of the 75 cases of lottery machine cycles. Consequently, the maximum error is reduced from 6% to 8%. Finally, at the last stage, the maximum error is reduced to 3% (balls with numbers 1, 4, and 10). Thus, with the increase in our sample the random error decreased. Theoretically, random errors could affect only one ball (or two balls), but the occurrence of each of the following random errors for the same ball is less and less probable (try to toss a coin – how many times will it fall on the same side?), while the occurrence of such errors for other balls is a more probable event. As a result, it turns out that the random errors tend to be mutually compensated.
But as we can see, random errors occurred at each of the four stages. At the first stage, the most frequently dropped ball is number 1, and the maximum deviation from the true value is 10%. At the second stage, the maximum random error is also observed for the ball with number 1, but becomes a little lower – 8%. At the third stage, the maximum error is observed for the ball with number 9, which dropped out in only 4% of the 75 cases of lottery machine cycles. Consequently, the maximum error is reduced from 6% to 8%. Finally, at the last stage, the maximum error is reduced to 3% (balls with numbers 1, 4, and 10). Thus, with the increase in our sample the random error decreased. Theoretically, random errors could affect only one ball (or two balls), but the occurrence of each of the following random errors for the same ball is less and less probable (try to toss a coin – how many times will it fall on the same side?), while the occurrence of such errors for other balls is a more probable event. As a result, it turns out that the random errors tend to be mutually compensated.
One of the main principles of the research in the frame of quantitative sociology is that applying different kinds of sample statistics distributions the sociologist can estimate the probability of obtaining the sample results due to random errors, i.e. to assess their possible impact on the results of the study.
Note the last line of the chart that shows the means for the sample at each stage. As you must remember from the previous chapters, the distribution of all possible sample means in this case corresponds to normal distribution. Consequently, obtaining a sample whose mean is close to the true mean (in our case it is equal to 5.5) is significantly higher than the probability of obtaining a sample whose mean is significantly different from the true mean. Based on the given data, the bigger our sample, the closer the sample mean is to the population mean. The difference between the sample mean and the population mean at each stage can also be interpreted as random errors. As you can see, at the last stage, with the sample of only 100 observations, the value of the random error is only 0.02.
Note the last line of the chart that shows the means for the sample at each stage. As you must remember from the previous chapters, the distribution of all possible sample means in this case corresponds to normal distribution. Consequently, obtaining a sample whose mean is close to the true mean (in our case it is equal to 5.5) is significantly higher than the probability of obtaining a sample whose mean is significantly different from the true mean. Based on the given data, the bigger our sample, the closer the sample mean is to the population mean. The difference between the sample mean and the population mean at each stage can also be interpreted as random errors. As you can see, at the last stage, with the sample of only 100 observations, the value of the random error is only 0.02.
- default_titleПаніотто В., Максименко В., Харченко Н. Статистичний аналіз соціологічних даних. - К.: Видавничий Дім «KM Академия», 2004.
- Show More