Manipulating the value of interval estimates; statistical significance and theoretical relevance
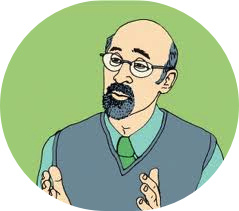
The connection between the width of the interval and the confidence level lies in the fact that the width of the interval is increased with the increase in the confidence level (i.e., error probability decreases as wider intervals have higher chances of including population value). Thus, the researchers themselves determine the alpha level or acceptable error probability (tolerance probability) (alpha is usually taken as 0.05 or lower). This applies to all methods of statistical conclusion.
Sample size is inversely related to the width of the interval. With the increase in the sample size the width of the interval decreases (at constant alpha level), i.e. larger samples provide more accurate estimates.
If we speak of statistical hypothesis testing, the probability of the null hypothesis deviation depends on four independent factors:
- НаблюдениеThe value of identified differences: this is the only factor that is not controlled by the researcher (and only partially depends on the specific measurement procedures).
- УчастиеAlpha value: the higher this value, the greater the critical domain and the easier it is to reject the null hypothesis.
- ИзмерениеTest type (one-tailed or two-tailed, see below): it is easier to reject the null hypothesis with the one-sided test.
- ИнтерегацияSample size: all other condition being equal, the larger the sample, the easier it is to reject the null hypothesis.
- Show More
Let us imagine that on the basis of a large sample (n > 1000) we detected the statistically significant difference between men (X mean = 1.4 hours per day) and women (X mean = 1.3 hours per day) in the amount of time spent on the Internet. This raises the question whether this difference between men and women is essential enough (0.1 hour or 6 minutes) to include it in the final report.
When statistical hypothesis testing is applied concerning the differences between groups (means and proportions), the researcher can choose between one-tailed and two-tailed test to determine the alpha level.
A one-tailed test is used when the researcher has the assumption about the direction of differences, that is, which group will have the greater prominence of the parameter in question.
If the researcher assumes that the groups differ, but is not sure which group will have the higher value, and which the lower, a two-tailed test is used.
Below the critical Z-values are presented (normal distribution) for both one-taled and two-tailed tests:
Alpha |
Z-value for a two-tailed test |
Z-value for a one-tailed test |
|
Left tail |
Right tail |
||
0,1 |
±1,65 |
-1,29 |
+1,29 |
0,05 |
±1,96 |
-1,65 |
+1,65 |
0,01 |
±2,58 |
-2,33 |
+2,33 |
0,001 |
±3,29 |
-3,10 |
+3,10 |
Let us assume the researcher compares men (X mean 1) and women (X mean 2) in the average amount of time they spend on the Internet. Let us say they determined alpha level of 0.01. Then, if they do not have any assumptions about which group spends more time on the Internet, they take the critical value of ± 2.58. The null hypothesis will be rejected if the empirical (or experimental) test value will be equal to or greater than 2.58 or equal to or lower than or -2.58.
If they believe that men spend more time on the Internet, then in order to reject the null hypothesis the empirical value must be equal to or greater than 2.33; if it is assumed that women devote more time to the Internet, then to reject the null hypothesis the empirical value must be equal to or lower than -2.33.
- default_titleХили Дж. Статистика. Социологические и маркетинговые исследования. - К.: ООО "ДиаСофтЮП"; СПб.: Питер, 2005. - 638 с.
- Show More